|
ACCESS THE FULL ARTICLE
No SPIE Account? Create one
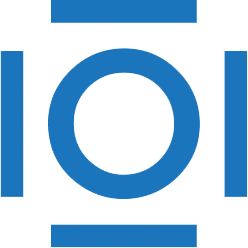
CITATIONS
Cited by 3 scholarly publications.
Lymphatic system
Image segmentation
Computed tomography
3D image processing
Detection and tracking algorithms
Medical imaging
Tissues