|
ACCESS THE FULL ARTICLE
No SPIE Account? Create one
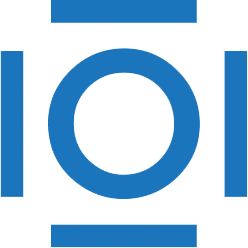
CITATIONS
Cited by 4 scholarly publications.
Image fusion
Vegetation
Airborne remote sensing
Roads
Visualization