|
ACCESS THE FULL ARTICLE
No SPIE Account? Create one
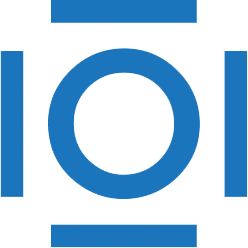
CITATIONS
Cited by 22 scholarly publications and 1 patent.
Scanning electron microscopy
Neural networks
Line width roughness
Denoising
Line edge roughness
Machine learning
Monte Carlo methods