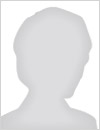
This will count as one of your downloads.
You will have access to both the presentation and article (if available).
When it is impossible or impractical to collect real labeled data, simulated data may be the only option. In active research being conducted by the Digital Imaging and Remote Sensing laboratory in the Chester F. Carlson Center for Imaging Science at the Rochester Institute of Technology, researchers are focusing on the estimation of the volume of condensed water vapor plumes that are generated from mechanical draft cooling towers, at a variety of facilities, using various modalities of remote sensing data from different imaging platforms. Prior research has supported the use of machine learning for plume segmentation and multi-view geometry techniques for three-dimensional reconstruction and subsequent volume estimation. To this point, real imagery has been collected from the ground and small unmanned aircraft systems with the end goal of exploring other potential collection platforms.
This research focuses on the training of a U-Net model to mask and segment these condensed water vapor plumes from other objects in the scene. The U-Net model and segmentation process has been successfully previously applied to real, low-altitude imagery and this research focuses on the use and application of the model through the use of simulated imagery. Several aspects of the simulation are of interest; how physically accurate do the scattering properties of the plume data need to be, how critical is the understanding of in situ meteorological conditions, how dependent is the process on the temporal and geographic variety in the data, how important is scene clutter and background type? The synthetic data used in this study was generated using the Digital Imaging and Remote Sensing Image Generation (DIRSIG) simulation environment and used to derive the inference and segmentation model to be tested on real imagery. While the trained artificial intelligence model performed consistently well when evaluated with synthetic imagery, the accuracy seen in the synthetic dataset did not translate into comparable results when evaluated with real imagery. However, the successes seen with the synthetic imagery and instances of real imagery results indicate that this binary classification and subsequent volume estimation is feasible to accomplish with high levels of accuracy in the future.
View contact details
No SPIE Account? Create one