|
ACCESS THE FULL ARTICLE
No SPIE Account? Create one
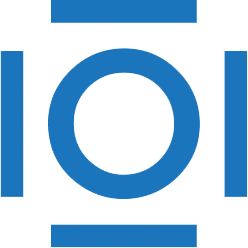
CITATIONS
Cited by 1 scholarly publication.
CAD systems
Computer aided diagnosis and therapy
Calibration
Computer aided design
Lung
Reliability
Classification systems