|
ACCESS THE FULL ARTICLE
No SPIE Account? Create one
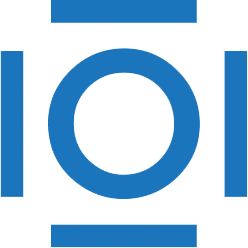
CITATIONS
Cited by 38 scholarly publications and 5 patents.
Tissues
Data modeling
Pathology
RGB color model
Prostate
Tumors
Visualization