|
ACCESS THE FULL ARTICLE
No SPIE Account? Create one
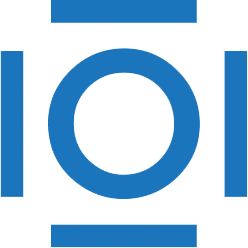
CITATIONS
Cited by 5 scholarly publications.
Image segmentation
Computed tomography
Brain
Neuroimaging
Convolutional neural networks
Head
Injuries