|
ACCESS THE FULL ARTICLE
No SPIE Account? Create one
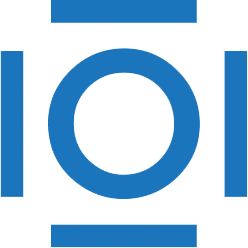
CITATIONS
Cited by 5 scholarly publications and 2 patents.
Computed tomography
Gallium nitride
Image quality
X-ray computed tomography
Head
Image quality standards
Neural networks