|
ACCESS THE FULL ARTICLE
No SPIE Account? Create one
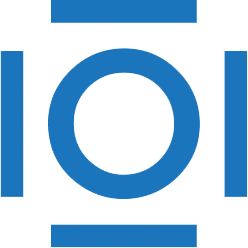
CITATIONS
Cited by 3 scholarly publications.
Breast
Computed tomography
Neural networks
Feature extraction
Image classification
Statistical analysis
Pattern recognition