|
ACCESS THE FULL ARTICLE
No SPIE Account? Create one
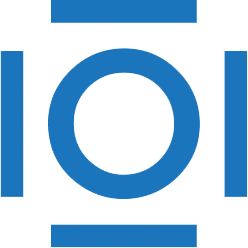
CITATIONS
Cited by 1 scholarly publication.
3D modeling
Virtual colonoscopy
Colon
Computed tomography
Data modeling
Network architectures
Colorectal cancer