|
ACCESS THE FULL ARTICLE
No SPIE Account? Create one
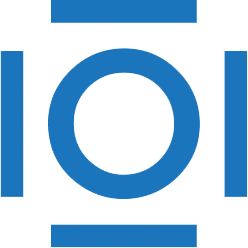
CITATIONS
Cited by 1 scholarly publication.
Lung
Chest imaging
Image segmentation
Opacity
Lung imaging
Oxygen
Pathology