|
ACCESS THE FULL ARTICLE
No SPIE Account? Create one
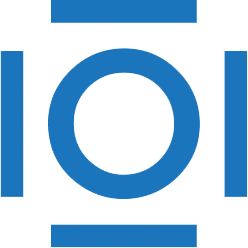
CITATIONS
Cited by 1 scholarly publication.
Feature selection
Machine learning
Data modeling
Error analysis
Statistical analysis
Statistical modeling
Algorithm development