|
ACCESS THE FULL ARTICLE
No SPIE Account? Create one
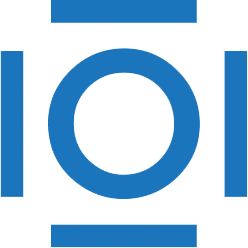
CITATIONS
Cited by 3 scholarly publications.
3D modeling
Computer aided diagnosis and therapy
3D image processing
Medical imaging
Performance modeling
Computed tomography
Virtual colonoscopy