|
ACCESS THE FULL ARTICLE
No SPIE Account? Create one
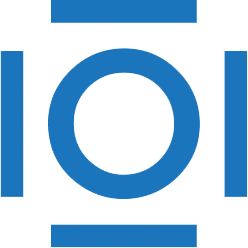
CITATIONS
Cited by 1 scholarly publication.
Cancer
Computer aided diagnosis and therapy
Virtual colonoscopy
Colorectal cancer
3D image processing
Radiology
Computed tomography