|
ACCESS THE FULL ARTICLE
No SPIE Account? Create one
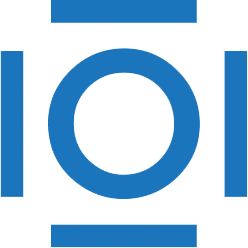
CITATIONS
Cited by 2 scholarly publications.
Network security
Information security
Defense and security
Computer security
Network architectures
Control systems
Defense technologies