|
ACCESS THE FULL ARTICLE
No SPIE Account? Create one
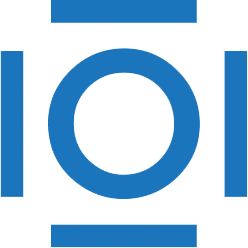
CITATIONS
Cited by 1 scholarly publication.
Magnetic resonance imaging
Convolutional neural networks
Image classification
Tumor growth modeling
Breast
Breast cancer
Computer aided diagnosis and therapy