|
ACCESS THE FULL ARTICLE
No SPIE Account? Create one
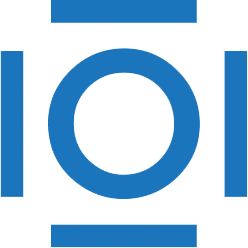
CITATIONS
Cited by 4 scholarly publications.
Sensors
Sensor fusion
Neurons
Data fusion
Neural networks
Binary data
Data modeling