|
ACCESS THE FULL ARTICLE
No SPIE Account? Create one
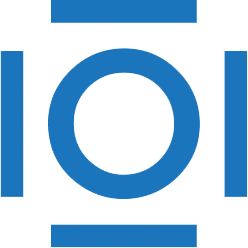
CITATIONS
Cited by 1 scholarly publication.
Image processing
Medical imaging
Digital image processing
Spatial resolution
Imaging systems
Image analysis
Image quality