|
ACCESS THE FULL ARTICLE
No SPIE Account? Create one
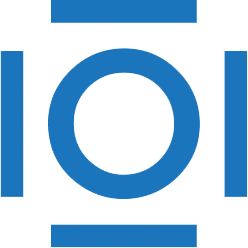
CITATIONS
Cited by 6 scholarly publications.
Scattering
Neural networks
Acoustics
Object recognition
Chlorine
Rayleigh scattering
Signal processing