|
ACCESS THE FULL ARTICLE
No SPIE Account? Create one
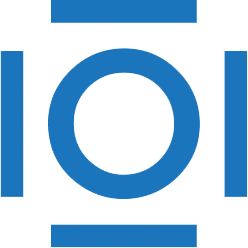
CITATIONS
Cited by 2 scholarly publications.
Neural networks
Network architectures
Error analysis
Fractal analysis
Sensors
Stochastic processes
Artificial neural networks