|
ACCESS THE FULL ARTICLE
No SPIE Account? Create one
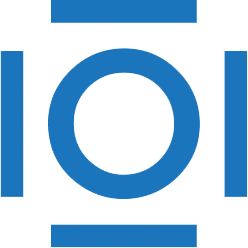
CITATIONS
Cited by 1 scholarly publication.
Neural networks
Agriculture
Image classification
Multispectral imaging
Data modeling
Earth observing sensors
Landsat