|
ACCESS THE FULL ARTICLE
No SPIE Account? Create one
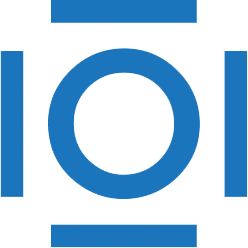
CITATIONS
Cited by 3 scholarly publications.
Prototyping
Fuzzy logic
Quantization
Statistical analysis
Detection and tracking algorithms
Probability theory
Control systems