|
ACCESS THE FULL ARTICLE
No SPIE Account? Create one
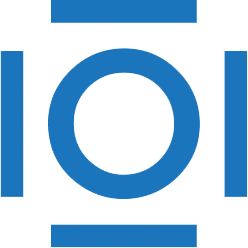
CITATIONS
Cited by 43 scholarly publications.
Stochastic processes
Image processing
Data modeling
Image analysis
Mathematical modeling
Optimization (mathematics)
Magnetorheological finishing