|
ACCESS THE FULL ARTICLE
No SPIE Account? Create one
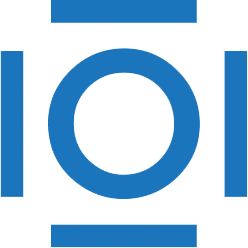
CITATIONS
Cited by 5 scholarly publications.
Neural networks
Image segmentation
Databases
Intelligence systems
Network architectures
Detection and tracking algorithms
Optical character recognition