|
ACCESS THE FULL ARTICLE
No SPIE Account? Create one
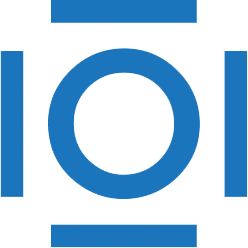
CITATIONS
Cited by 3 scholarly publications.
Pattern recognition
Neural networks
Damage detection
Image classification
Nondestructive evaluation
Performance modeling
Finite element methods