|
ACCESS THE FULL ARTICLE
No SPIE Account? Create one
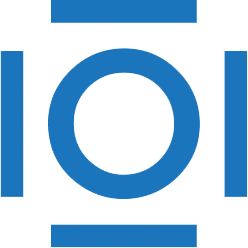
CITATIONS
Cited by 3 scholarly publications.
Signal to noise ratio
Digital filtering
Linear filtering
Optical filters
Electronic filtering
Statistical analysis
Optimal filtering