|
ACCESS THE FULL ARTICLE
No SPIE Account? Create one
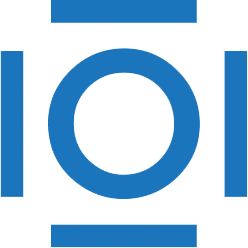
CITATIONS
Cited by 1 scholarly publication.
Image compression
Neural networks
Image quality
Image processing
Quantization
Computer simulations
Neurons