|
ACCESS THE FULL ARTICLE
No SPIE Account? Create one
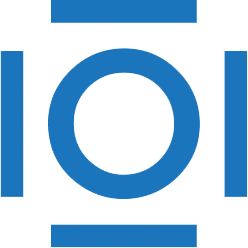
CITATIONS
Cited by 5 scholarly publications.
Wavelets
Image compression
Image filtering
Mammography
Prototyping
Quantization
Filtering (signal processing)