|
ACCESS THE FULL ARTICLE
No SPIE Account? Create one
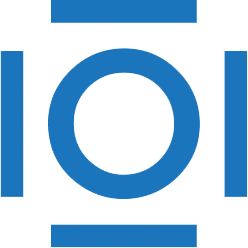
CITATIONS
Cited by 54 scholarly publications.
Sensors
Filtering (signal processing)
Data fusion
Navigation systems
Data modeling
Kinematics
Mars