|
ACCESS THE FULL ARTICLE
No SPIE Account? Create one
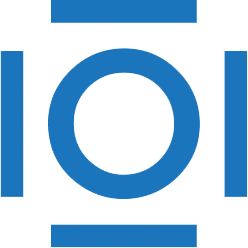
CITATIONS
Cited by 3 scholarly publications.
Sensors
3D modeling
Data modeling
Target detection
Detection and tracking algorithms
Hyperspectral imaging
Hyperspectral target detection