|
ACCESS THE FULL ARTICLE
No SPIE Account? Create one
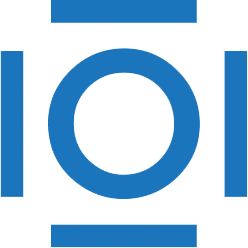
CITATIONS
Cited by 3 scholarly publications.
Stochastic processes
Monte Carlo methods
Control systems
Sensors
Target detection
Detection and tracking algorithms
Statistical analysis