|
ACCESS THE FULL ARTICLE
No SPIE Account? Create one
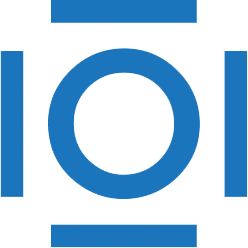
CITATIONS
Cited by 10 scholarly publications.
Nonlinear filtering
Signal to noise ratio
Radar
Motion models
Algorithm development
Detection and tracking algorithms
Data modeling