|
ACCESS THE FULL ARTICLE
No SPIE Account? Create one
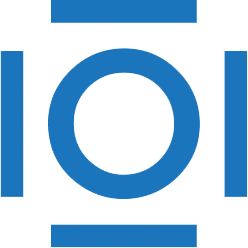
CITATIONS
Cited by 9 scholarly publications.
Data modeling
Inverse problems
Probability theory
Bayesian inference
Optimization (mathematics)
Radon
Lead