|
ACCESS THE FULL ARTICLE
No SPIE Account? Create one
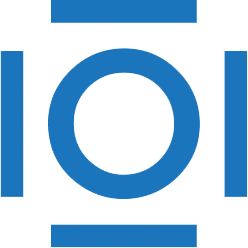
CITATIONS
Cited by 2 scholarly publications.
Feature extraction
Neural networks
Pattern recognition
Neurons
Analog electronics
Binary data
Electroluminescence