|
ACCESS THE FULL ARTICLE
No SPIE Account? Create one
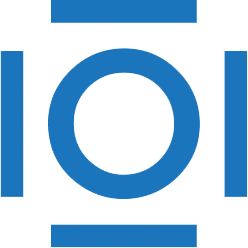
CITATIONS
Cited by 3 scholarly publications.
Synthetic aperture radar
Image classification
Neural networks
Error analysis
Image resolution
Prototyping
Sensors