|
ACCESS THE FULL ARTICLE
No SPIE Account? Create one
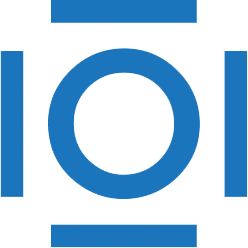
CITATIONS
Cited by 35 scholarly publications.
Synthetic aperture radar
Virtual colonoscopy
Automatic target recognition
Binary data
Data acquisition
Fourier transforms
MATLAB