|
ACCESS THE FULL ARTICLE
No SPIE Account? Create one
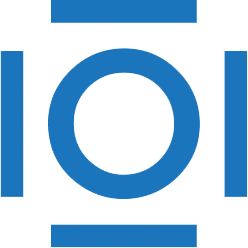
CITATIONS
Cited by 8 scholarly publications.
Wavelets
Deconvolution
Interference (communication)
Convolution
Distortion
Filtering (signal processing)
Signal to noise ratio