|
ACCESS THE FULL ARTICLE
No SPIE Account? Create one
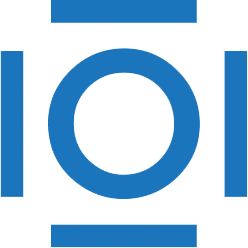
CITATIONS
Cited by 1 scholarly publication.
Data modeling
Neural networks
Unexploded object detection
Coastal modeling
Eye
Eye models
Analytical research