|
ACCESS THE FULL ARTICLE
No SPIE Account? Create one
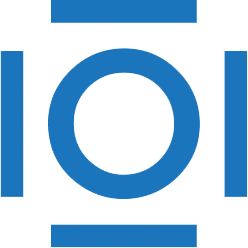
CITATIONS
Cited by 4 scholarly publications.
Computer simulations
Monte Carlo methods
Data modeling
Sensors
Instrument modeling
Device simulation
Thermal modeling