|
ACCESS THE FULL ARTICLE
No SPIE Account? Create one
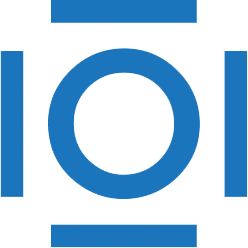
CITATIONS
Cited by 1 scholarly publication.
Hyperspectral imaging
Stochastic processes
Spectral models
Data hiding
Image analysis
Model-based design
Silicon