|
ACCESS THE FULL ARTICLE
No SPIE Account? Create one
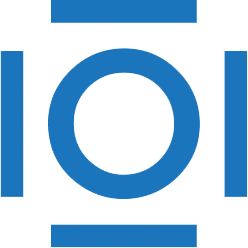
CITATIONS
Cited by 6 scholarly publications.
Signal detection
Mammography
Computer aided diagnosis and therapy
Neural networks
Feature extraction
Electronic filtering
Analytical research