|
ACCESS THE FULL ARTICLE
No SPIE Account? Create one
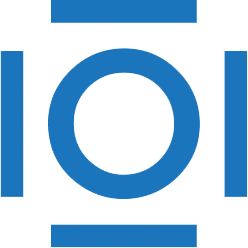
CITATIONS
Cited by 33 scholarly publications.
Object recognition
Target detection
Visual process modeling
Visualization
Detection and tracking algorithms
Computing systems
Machine vision