|
ACCESS THE FULL ARTICLE
No SPIE Account? Create one
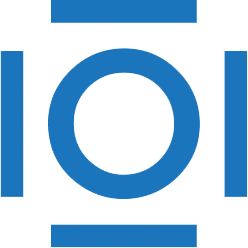
CITATIONS
Cited by 3 scholarly publications.
Expectation maximization algorithms
Principal component analysis
Image classification
Remote sensing
Multispectral imaging
Statistical analysis
Earth observing sensors