|
ACCESS THE FULL ARTICLE
No SPIE Account? Create one
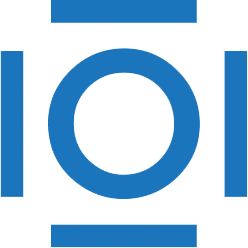
CITATIONS
Cited by 2 scholarly publications.
Principal component analysis
Feature selection
Databases
Feature extraction
Inspection
Automatic target recognition
Detection and tracking algorithms