|
ACCESS THE FULL ARTICLE
No SPIE Account? Create one
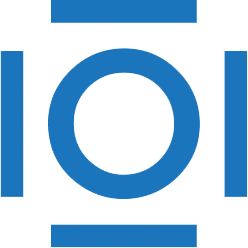
CITATIONS
Cited by 1 scholarly publication and 2 patents.
Ferroelectric materials
Bismuth
Picosecond phenomena
Information technology
Weapons of mass destruction
Signal processing