|
ACCESS THE FULL ARTICLE
No SPIE Account? Create one
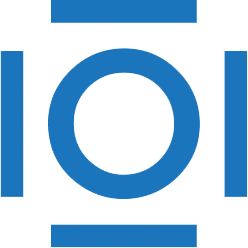
CITATIONS
Cited by 19 scholarly publications and 3 patents.
Computer aided diagnosis and therapy
Lung
Artificial neural networks
Cancer
Lung cancer
Computer aided design
Databases