|
ACCESS THE FULL ARTICLE
No SPIE Account? Create one
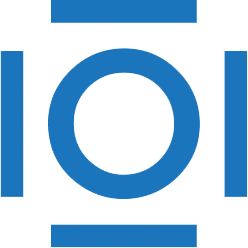
CITATIONS
Cited by 1 scholarly publication.
Condition numbers
Hyperspectral imaging
Projection systems
Error analysis
Interference (communication)
Linear algebra
Principal component analysis