|
ACCESS THE FULL ARTICLE
No SPIE Account? Create one
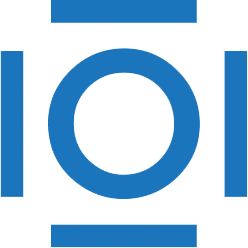
CITATIONS
Cited by 3 scholarly publications.
Neural networks
Knowledge discovery
Principal component analysis
Feature selection
Data modeling
Absorption
Reflectivity