|
ACCESS THE FULL ARTICLE
No SPIE Account? Create one
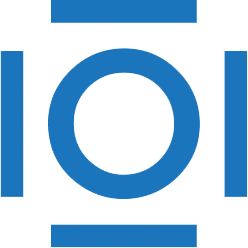
CITATIONS
Cited by 5 scholarly publications.
Quantization
Lymphoma
Cancer
Feature selection
Genetic algorithms
Tumors
Visualization