|
ACCESS THE FULL ARTICLE
No SPIE Account? Create one
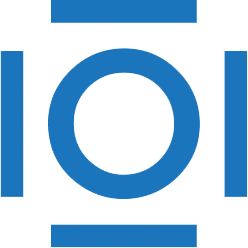
CITATIONS
Cited by 1 scholarly publication.
Lawrencium
Error analysis
Artificial neural networks
Computer aided diagnosis and therapy
Statistical analysis
Analytical research
Receivers